- Daily Dose of Data Science
- Posts
- Momentum: Explained Visually and Intuitively
Momentum: Explained Visually and Intuitively
Speedup machine learning model training with little effort.
As we progress towards building larger and larger models, every bit of possible optimization becomes crucial.

And, of course, there are various ways to speed up model training, like:
Leverage distributed training using frameworks like PySpark MLLib.
Use better Hyperparameter Optimization, like Bayesian Optimization, which we discussed here: Bayesian Optimization for Hyperparameter Tuning.
or the 15 different techniques we covered here: 15 Ways to Optimize Neural Network Training (With Implementation).
Momentum is another reliable and effective technique to speed up model training that I missed covering in those 15 techniques.
While it is pretty popular, today, I want to share an intuitive guide that dives into how it works and why it is effective.
Let’s begin!
Issues with Gradient Descent
In gradient descent, every parameter update solely depends on the current gradient.
This is clear from the gradient weight update rule shown below:

As a result, we end up having many unwanted oscillations during the optimization process.
Let’s understand this more visually.
Imagine this is the loss function contour plot, and the optimal location (parameter configuration where the loss function is minimum) is marked here:

Simply put, this plot illustrates how gradient descent moves towards the optimal solution. At each iteration, the algorithm calculates the gradient of the loss function at the current parameter values and updates the weights.
This is depicted below:

Notice two things here:
It unnecessarily oscillates vertically.
It ends up at the non-optimal solution after some epochs.
Ideally, we would have expected our weight updates to look this:

It must have taken longer steps in the horizontal direction…
…and smaller vertical steps because a movement in this direction is unnecessary.
This idea is also depicted below:
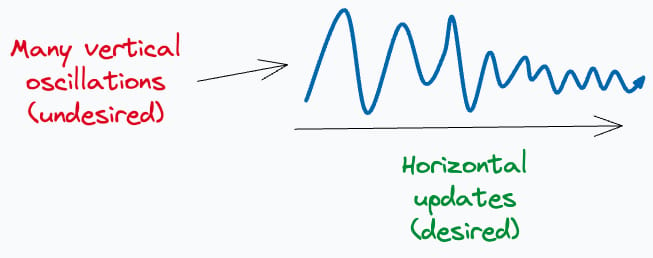
How Momentum solves this problem?
Momentum-based optimization slightly modifies the update rule of gradient descent.
More specifically, it also considers a moving average of past gradients:

This helps us handle the unnecessary vertical oscillations we saw earlier.
How?
As Momentum considers a moving average of past gradients, so if the recent gradient update trajectory looks as shown in the following image, then it is clear that its average in the vertical direction will be very low while that in the horizontal direction will be large (which is precisely what we want):

As this moving average gets added to the gradient updates, it helps the optimization algorithm take larger steps in the desired direction.

This way, we can:
Smoothen the optimization trajectory.
Reduce unnecessary oscillations in parameter updates, which also speeds up training.
This is also evident from the image below:

This time, the gradient update trajectory shows much smaller oscillations in the vertical direction, and it also manages to reach an optimum under the same number of epochs as earlier.
This is the core idea behind Momentum and how it works.
Of course, Momentum does introduce another hyperparameter (Momentum rate) in the model, which should be tuned appropriately like any other hyperparameter:

For instance, considering the 2D contours we discussed above:
Setting an extremely large value of Momentum rate will significantly expedite gradient update in the horizontal direction. This may lead to overshooting the minima, as depicted below:

What’s more, setting an extremely small value of Momentum will slow down the optimal gradient update, defeating the whole purpose of Momentum.

This animation summarizes the usage of momentum:

👉 Over to you: What are some other reliable ways to speed up machine learning model training?
We covered 15 different techniques here: 15 Ways to Optimize Neural Network Training (With Implementation).
If you want to have a more hands-on experience, check out this tool: Momentum Tool.
For those wanting to develop “Industry ML” expertise:
All businesses care about impact.
Can you reduce costs?
Drive revenue?
Can you scale ML models?
Predict trends before they happen?
We have discussed several other topics (with implementations) that align with “Industry ML.” Here are some of them:
Learn sophisticated graph architectures and how to train them on graph data: A Crash Course on Graph Neural Networks – Part 1
Learn techniques to run large models on small devices: Quantization: Optimize ML Models to Run Them on Tiny Hardware
Learn how to generate prediction intervals or sets with strong statistical guarantees for increasing trust: Conformal Predictions: Build Confidence in Your ML Model's Predictions.
Learn how to identify causal relationships and answer business questions: A Crash Course on Causality – Part 1
Learn how to scale ML model training: A Practical Guide to Scaling ML Model Training.
Learn techniques to reliably roll out new models in production: 5 Must-Know Ways to Test ML Models in Production (Implementation Included)
Learn how to build privacy-first ML systems: Federated Learning: A Critical Step Towards Privacy-Preserving Machine Learning.
Learn how to compress ML models and reduce costs: Model Compression: A Critical Step Towards Efficient Machine Learning.
Being able to code is a skill that’s diluting day by day.
Thus, the ability to make decisions, guide strategy, and build solutions that solve real business problems and have a business impact will separate practitioners from experts.
SPONSOR US
Get your product in front of ~90,000 data scientists and other tech professionals.
Our newsletter puts your products and services directly in front of an audience that matters — thousands of leaders, senior data scientists, machine learning engineers, data analysts, etc., who have influence over significant tech decisions and big purchases.
To ensure your product reaches this influential audience, reserve your space here or reply to this email to ensure your product reaches this influential audience.
Reply