- Daily Dose of Data Science
- Posts
- A Popular Interview Question: Explain Discriminative and Generative Models
A Popular Interview Question: Explain Discriminative and Generative Models
A simplified guide to generative and discriminative models, along with a quiz.
Many machine learning models can be classified into two categories:
Generative
Discriminative
Understanding this difference is critical because it sheds light on the fundamental approach each type of model takes in solving a given problem.
This will make it easier for you to figure out which route aligns better with the characteristics of your dataset.
The following visual depicts how they differ:

Today, let’s understand what they are in detail.
#1) Discriminative models

Discriminative models, as the name suggests, are primarily centered around learning decision boundaries that separate different classes.
Mathematically speaking, they maximize the conditional probability P(Y|X), which is read as follows: “Given an input X, maximize the probability of label Y.”
As a result, these types of models are explicitly meant for classification tasks.
Popular examples include:
Logistic regression
Random Forest
Neural Networks
Decision Trees, etc.
#2) Generative models

Generative models, on the other hand, are primarily centered around learning the class-conditional distribution, as shown in the figure above.
Thus, they maximize the joint probability P(X, Y) by learning the class-conditional distribution P(X|Y):

Popular examples include:
Naive Bayes
Linear Discriminant Analysis (LDA)
Gaussian Mixture Models, etc.
We covered Joint and Conditional probability before. Read this post if you wish to learn what they are: A Visual Guide to Joint, Marginal and Conditional Probabilities.
As generative models learn the underlying distribution, they can generate new samples.

However, this is not possible with discriminative models.
Furthermore, generative models possess discriminative properties, i.e., they can be used for classification tasks (if needed).

However, discriminative models do not possess generative properties.
Discriminative vs. Generative Quiz
Let’s consider an example to better understand them.
Imagine you are a language classification system.

There are two ways you can classify languages.
Learn every language and then classify a new language based on acquired knowledge.
Understand some distinctive patterns in each language without truly learning the language. Once you do that, classify a new language based on the learned patterns.
Can you figure out which of the above is generative and which is discriminative?
Answer
The first approach is generative. This is because you learned the underlying distribution of each language.
In other words, you learned the joint distribution P(Words, Language).

Moreover, as you understand the underlying distribution, now you can generate new sentences, can’t you?
The second approach is discriminative. This is because you only learned specific distinctive patterns of each language.
It is like:
If so and so words appear, it is likely “Langauge A.”
If this specific set of words appears, it is likely “Langauge B.”
and so on.

In other words, you learned the conditional distribution P(Language|Words).
Can you generate new sentences here?
No, right?
This is the difference between generative and discriminative models.
Also, the above description might persuade you that generative models are more generally useful, but it is not true.
This is because generative models have their own modeling complications.
For instance, typically, generative models require more data than discriminative models.
Relate it to the language classification example again.
Imagine the amount of data you would need to learn all languages (generative approach) vs. the amount of data you would need to understand some distinctive patterns (discriminative approach).
Typically, discriminative models outperform generative models in classification tasks.
Discriminative models can be further categorized into two categories, which we shall learn in tomorrow’s post.
👉 Till then, it’s Over to you: What are some other problems while training generative models?
👉 If you liked this post, don’t forget to leave a like ❤️. It helps more people discover this newsletter on Substack and tells me that you appreciate reading these daily insights.
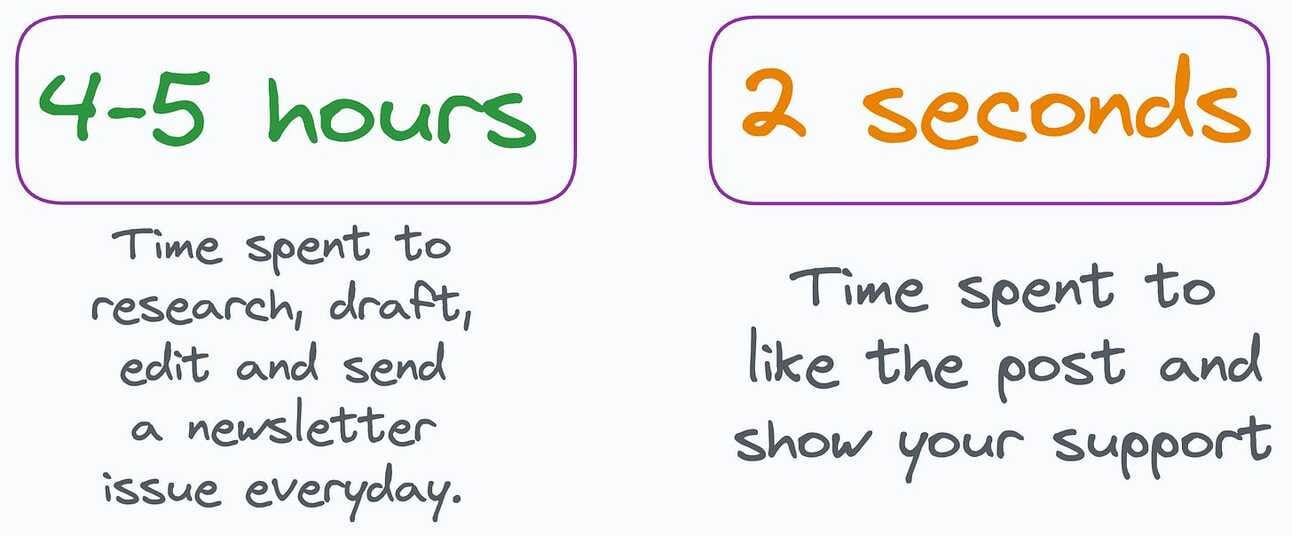
The button is located towards the bottom of this email.
Thanks for reading!
Latest full articles
If you’re not a full subscriber, here’s what you missed last month:
Don’t Stop at Pandas and Sklearn! Get Started with Spark DataFrames and Big Data ML using PySpark.
DBSCAN++: The Faster and Scalable Alternative to DBSCAN Clustering
Federated Learning: A Critical Step Towards Privacy-Preserving Machine Learning
You Cannot Build Large Data Projects Until You Learn Data Version Control!
Deploy, Version Control, and Manage ML Models Right From Your Jupyter Notebook with Modelbit
To receive all full articles and support the Daily Dose of Data Science, consider subscribing:
👉 Tell the world what makes this newsletter special for you by leaving a review here :)
👉 If you love reading this newsletter, feel free to share it with friends!
Reply